Building Trust in AI: Transparency, Accountability, and Explainability
- MOHD EZLI MASHUT
- May 5, 2024
- 4 min read
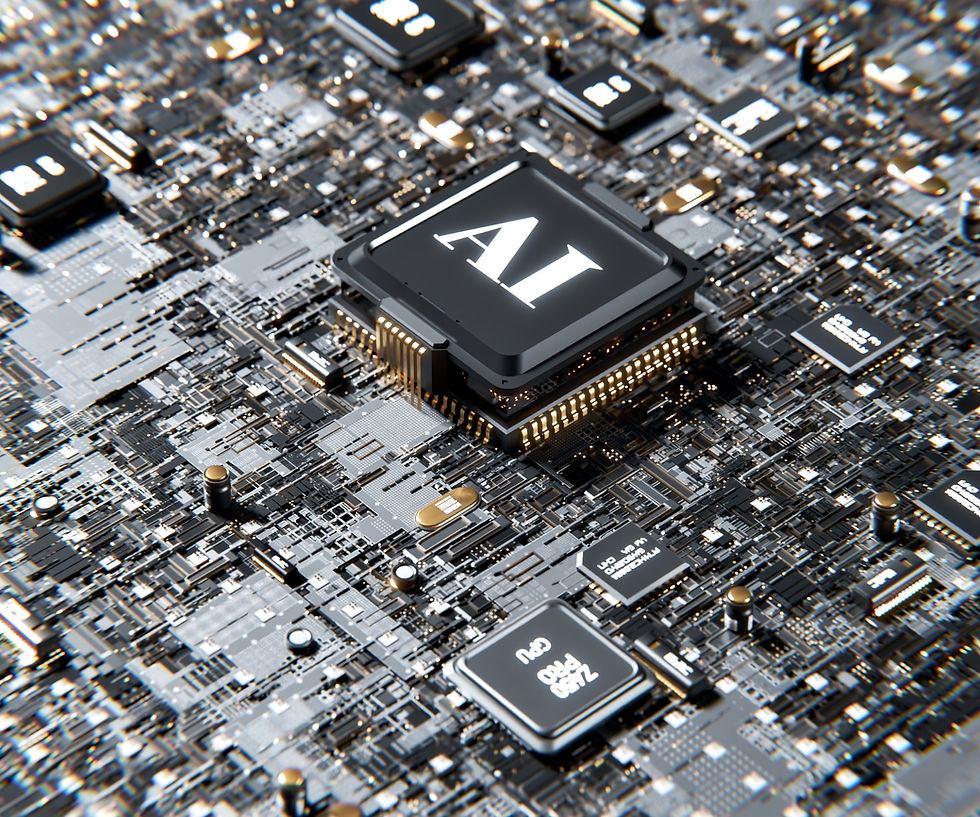
BY : Mohd Ezli Mashut & ChatGPT
In an era where artificial intelligence (AI) permeates various aspects of our lives, from personalized recommendations on streaming platforms to healthcare diagnostics, the need for trust in AI systems has become paramount.
Trust forms the bedrock of any successful relationship, whether between individuals or between humans and technology.
However, establishing trust in AI is not a straightforward task. It requires addressing complex issues such as transparency, accountability, and explainability.
In this comprehensive exploration, we delve into the intricacies of building trust in AI systems. We will examine the significance of transparency, the importance of accountability mechanisms, and the necessity of explainability in AI algorithms.
Furthermore, we will discuss strategies to overcome trust and privacy issues, safeguarding the integrity of AI technologies.
The Importance of Trust in AI
Before delving into the mechanisms to build trust in AI, it's crucial to understand why trust is indispensable in this domain. AI algorithms wield considerable influence over our lives, shaping decisions ranging from loan approvals to hiring processes. Without trust, users may hesitate to adopt AI-powered solutions, leading to skepticism and resistance. Trust fosters acceptance and adoption, paving the way for the ethical and responsible deployment of AI technologies.
Transparency: Shedding Light on AI Systems
Transparency lies at the heart of building trust in AI. It entails openness and clarity regarding the workings of AI algorithms, data sources, and decision-making processes. When users understand how AI systems operate, they are more likely to trust their outcomes. Transparency also facilitates accountability, allowing stakeholders to scrutinize AI systems for biases, errors, or unethical practices.
Achieving transparency in AI involves several key practices:
1. Algorithmic Transparency: AI developers should disclose information about the algorithms used in their systems, including their objectives, inputs, and decision-making logic. This transparency enables users to comprehend how AI arrives at its conclusions.
2. Data Transparency: Transparency regarding data sources, collection methods, and preprocessing techniques is essential for building trust in AI. Users should have visibility into the types of data used to train AI models and any biases present in the dataset.
3. Model Performance Metrics: Providing metrics such as accuracy, precision, recall, and fairness metrics offers insights into the performance of AI models. Transparent reporting of these metrics helps users evaluate the reliability and effectiveness of AI systems.
Accountability: Holding AI Systems Responsible
Accountability mechanisms are crucial for ensuring that AI systems operate ethically and responsibly. Accountability involves holding AI developers, deployers, and users answerable for the outcomes of AI technologies. Establishing clear lines of accountability promotes trust by fostering confidence that actions will have consequences.
Key elements of accountability in AI include:
1. Regulatory Frameworks: Governments and regulatory bodies play a vital role in establishing laws and guidelines to govern the use of AI. These regulations outline ethical standards, data privacy protections, and mechanisms for accountability, providing a framework for responsible AI deployment.
2. Ethical Guidelines: AI developers should adhere to ethical guidelines and principles that prioritize fairness, transparency, and user welfare. Ethical AI frameworks, such as the IEEE Ethically Aligned Design, offer guidance on designing and deploying AI systems in a responsible manner.
3. Internal Controls: Organizations deploying AI systems should implement internal controls and oversight mechanisms to monitor AI operations, detect biases or errors, and ensure compliance with ethical standards. This includes conducting regular audits and assessments of AI systems' performance and impact.
Explainability: Making AI Understandable
Explainability refers to the ability of AI systems to provide understandable explanations for their decisions and predictions. Explainable AI (XAI) is essential for building trust by demystifying the black-box nature of complex algorithms. When users can comprehend the reasoning behind AI decisions, they are more likely to trust and accept its outcomes.
Key strategies for achieving explainability in AI include:
1. Interpretability Techniques: Employing interpretable machine learning models, such as decision trees or linear models, can enhance the explainability of AI systems. These models offer transparent decision-making processes that users can easily understand.
2. Post-hoc Explanation Methods: Post-hoc explanation techniques, such as LIME (Local Interpretable Model-Agnostic Explanations) or SHAP (SHapley Additive exPlanations), provide insights into individual predictions by highlighting the features that influenced the model's output. These methods offer transparent explanations for AI decisions, increasing trust and comprehension.
3. Human-AI Collaboration: Facilitating human-AI collaboration allows users to interact with AI systems to seek explanations for their decisions. Chatbots, conversational interfaces, and interactive visualizations enable users to query AI systems and receive understandable explanations in natural language.
Overcoming Trust and Privacy Issues in AI
While transparency, accountability, and explainability are essential pillars for building trust in AI, addressing trust and privacy issues requires a multifaceted approach. Here are some strategies to overcome these challenges:
1. Data Privacy Protection: Implement robust data privacy measures, such as anonymization, encryption, and access controls, to safeguard sensitive information and mitigate privacy risks associated with AI systems.
2. Bias Detection and Mitigation: Employ bias detection techniques to identify biases present in AI algorithms or datasets. Mitigate biases through techniques such as data augmentation, algorithmic adjustments, or diversification of training data to ensure fairness and equity.
3. User Education and Engagement: Educate users about the capabilities and limitations of AI systems to manage expectations and foster informed decision-making. Encourage user feedback and engagement to iteratively improve AI algorithms and enhance user trust.
4. Third-party Audits and Certification: Engage third-party auditors or certification bodies to assess the fairness, transparency, and accountability of AI systems. Independent audits provide assurance to stakeholders and validate compliance with ethical standards.
Conclusion
Building trust in AI is a multifaceted endeavor that requires transparency, accountability, and explainability. By embracing these principles and implementing strategies to overcome trust and privacy issues, we can foster confidence in AI technologies and ensure their ethical and responsible use.
As AI continues to shape our world, cultivating trust is essential for harnessing its transformative potential while safeguarding the interests and well-being of society.
In conclusion, the journey toward trustworthy AI is ongoing, but by prioritizing transparency, accountability, and explainability, we can pave the way for a future where AI technologies inspire confidence, empower users, and drive positive societal impact.
Comentarios